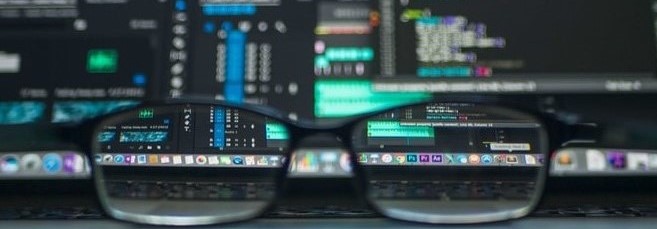
14 May What is machine learning?
What is Machine Learning?
A lot has been made of the transformative potential of artificial intelligence (AI), and for good reason. As the most advanced form of artificial intelligence, machine learning is shaping up to be nothing short of a revolution as we all scramble to implement it’s super smart capabilities into the enterprise.
Powered by advances in processing power, big data, and next-gen algorithms, it finally feels as if machine learning will come to practical fruition fairly soon. So, it is no surprise to hear that IDC is predicting the marketplace for AI and machine learning tech will approach $98.4 billion in revenue through 2023.
While the excitement is warranted, there’s still some confusion in the marketplace around exactly what machine learning is and isn’t. An easy definition paints machine learning as an advanced application of AI that provides intelligent machines with access to data and lets them teach themselves. Is this the singularity? Are the days of human beings numbered?
Machines will replace humans in many aspects of decision making, but machine learning will always have some margin of error because it ultimately operates on degrees of probability. By trawling through large amounts of data, it can make predictions and recommendations to a relative degree of certainty, but it will still need humans to put it on the right track.
The actual “learning” aspect is a kind of feedback loop where decisions are deemed correct or incorrect. This correction can be done by humans, or another algorithm, but the corrections are what enables it to make better future predictions.
As we know with any probability calculation, the larger the amount of data we can pull from, the greater the accuracy will be. Where previous intelligence systems struggled to cope with the massive digital data sets available in an enterprise, machine learning applications actually thrive on these large volumes of data. Data scientists can move beyond using only a sample set of data, to using every possible detail contained within the entire data set for uncovering real evidence.
What do we need to focus on to make it work?
We shouldn’t get ahead of ourselves though, as machine learning won’t just chew and digest any old data you throw at it. Machine learning applications and their outputs will only be as valuable as the quality of the data provided to them. This means organisations will first need to undertake a few key steps in their digital transformation including:
- understanding how their data is collected
- where the data is stored and who has access
- what state the data is in currently in terms of quality
- how they can integrate and merge data from silos across the organisation
But this critical step of capturing, preparing, and analysing data, in turn creates the foundation for successful machine learning initiatives. In terms of where these applications should first be deployed, we suggest starting small and using successful use cases as evidence for further investment.
Key questions to ask yourself?
Focus on those processes that are most commonly used across your organisation, as these are the areas that will provide the greatest business value for machine learning. At a broad level, ask yourself:
- Where are the most unstructured work patterns?
- Where could we see real productivity gains from machine learning?
- What are the customer’s common pain points that could be improved?
The ideal use cases are those which have large historical data sets that can be used to build an algorithm for decreasing operational costs or increasing revenue. This is where competitive advantage can be generated, but don’t forget – you can’t learn anything without good quality data.
Michael Power | 14 May 2020